A Strategic Roadmap to AI Operationalization
AI represents arguably the most transformative technological innovation of the modern era. Although AI has the potential to uncover previously unimaginable and unexplored opportunities for growth, optimization, monetization, and differentiation for enterprises of all sizes across industries, numerous significant challenges inhibit the ability to fully realize the technology’s full value potential. Many of these challenges stem from the lack of a highly robust, detailed and deliberate AI operationalization roadmap strategy, which only adds to the complexity, difficulty and intricacy of effective AI development, implementation and operation. Thus, by developing a tailored, tangible and strategic roadmap that accounts for the specific and nuanced challenges encountered with initiatives, enterprises can bolster their AI posture, maturity and ecosystem to better enable effective and efficient AI operationalization and value realization.
AI Operationalization Challenges
Enterprises face significant challenges in realizing the full benefits of AI initiatives due to the lack of a mature, supportive and enabled AI posture and ecosystem. Given the inherent complexity of AI technologies and the accelerating dynamics impacting their development, many AI projects have failed to yield the value and intended impact expected by organizations and executives. To more effectively capture the transformative business value offered by AI technologies, enterprises must prioritize operational, technical, and governance initiatives designed specifically to address the following leading challenges to AI operationalization.
Enterprise Readiness & Enablement
The Vation Ventures 2024 Technology Executive Outlook Report revealed that over 60% of global CXOs from across industries prioritize AI, particularly for enhancing productivity and implementing responsible AI practices. Despite AI being recognized by 96% of executives as the most impactful near-term technology, most organizations are still in the nascent stages of AI maturity. More than 60% of enterprises are in the initial awareness or planning phases, highlighting a significant lag in execution and the opportunity to ensure effective operationalization.
.png)
For enterprises that have undertaken AI initiatives, there is a notable gap between the expected benefits of initiatives and their actual realization, often stemming from a lack of a fully mature and supportive AI ecosystem within enterprises. This maturity gap can lead to AI projects falling short of their goals, particularly in generating enhanced operational productivity, efficiency, and speed. In a rush to capitalize on the potential of AI and secure a competitive edge, many enterprises fail to adequately plan, map and account for the challenges, resourcing, requirements and impacts of AI initiatives. As a result, the lack of systematic preparation, understanding and alignment can significantly limit the ability of initiatives to meet expectations and deliver value.

One of the more significant AI operationalization challenges has to do with the difficulty of measuring and demonstrating the business value of AI, which remains a critical barrier to widespread AI adoption. Often, organizations find it challenging to establish clear metrics and KPIs that accurately capture the impact of AI projects. This challenge is compounded by the lack of standardized and meaningful benchmarking and the complexity of AI systems, which may produce indirect or intangible benefits that are hard to quantify. Without clear metrics, it becomes difficult for businesses to justify ongoing investment in AI technologies, leading to stalled or abandoned projects.
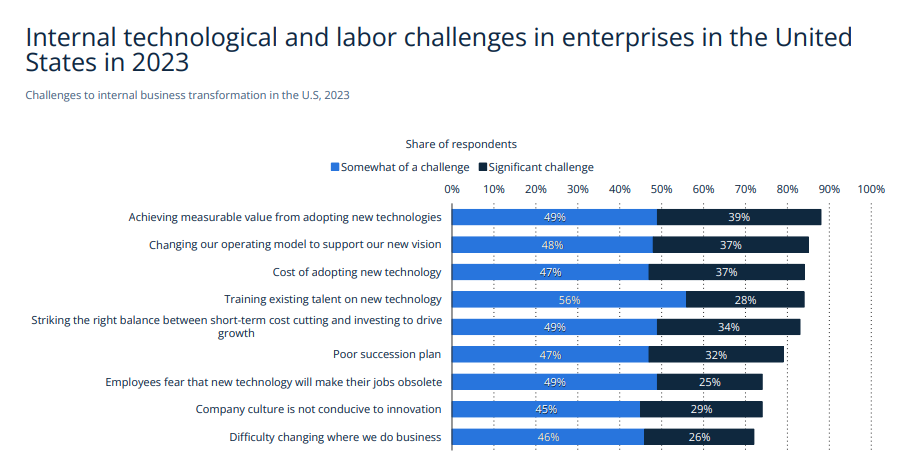
Technical Talent
Another challenge hindering the achievement of a mature and robust enterprise AI posture and ecosystem is the widespread technical talent gap. The Vation Ventures’ 2024 Technology Executive Outlook Report found that 72% of organizations identified AI as their most critical talent deficit, more than double that of the second largest talent gap. Further, a shortage of skilled talent was identified as the third largest and most frequent challenge to AI initiative implementation, reinforcing the growing foundational importance of securing and developing technical talent and enablement to ensure effective operationalization and utilization.
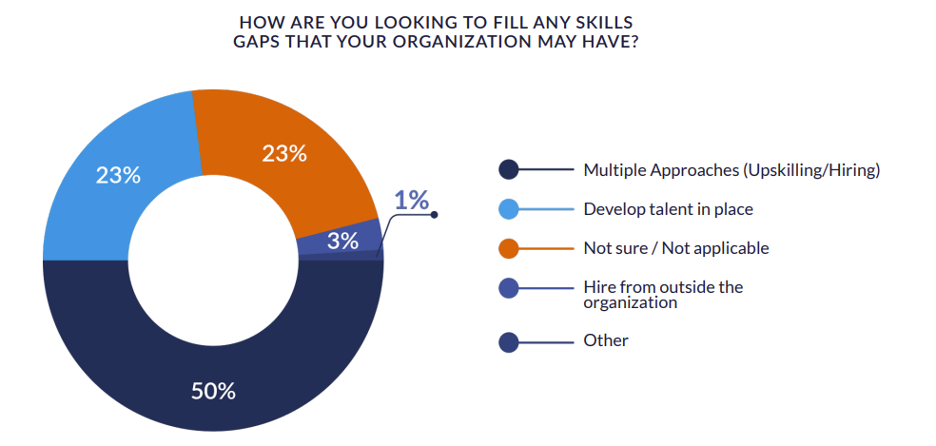
The technical talent gap underscores a pressing need for comprehensive strategies that include developing internal expertise, investing in advanced enablement tooling and fostering a culture of continuous learning. According to the Vation Ventures global community of CXOs, 50% of organizations intend to utilize a hybrid upskilling and hiring approach to address their talent gaps, underscoring the need for specialized talent and general, enterprise-wide training and enablement. Without addressing these foundational issues, enterprises will struggle to move beyond the planning stages and fully capitalize on AI’s transformative potential.
Data Maturity
Data maturity has become a critical cornerstone for successful AI operationalization in the rapidly evolving technological and competitive landscape. The increasing complexity of managing ever-expanding data ecosystems and the growing need for operational agility underscores the importance of mature data management practices. Effective data maturity involves ensuring data quality, consistency and accessibility, which are essential for training accurate and reliable AI models. As AI applications grow more sophisticated and data-intensive, the ability to manage and process large volumes of data efficiently will increasingly serve as a competitive differentiator for enterprises.
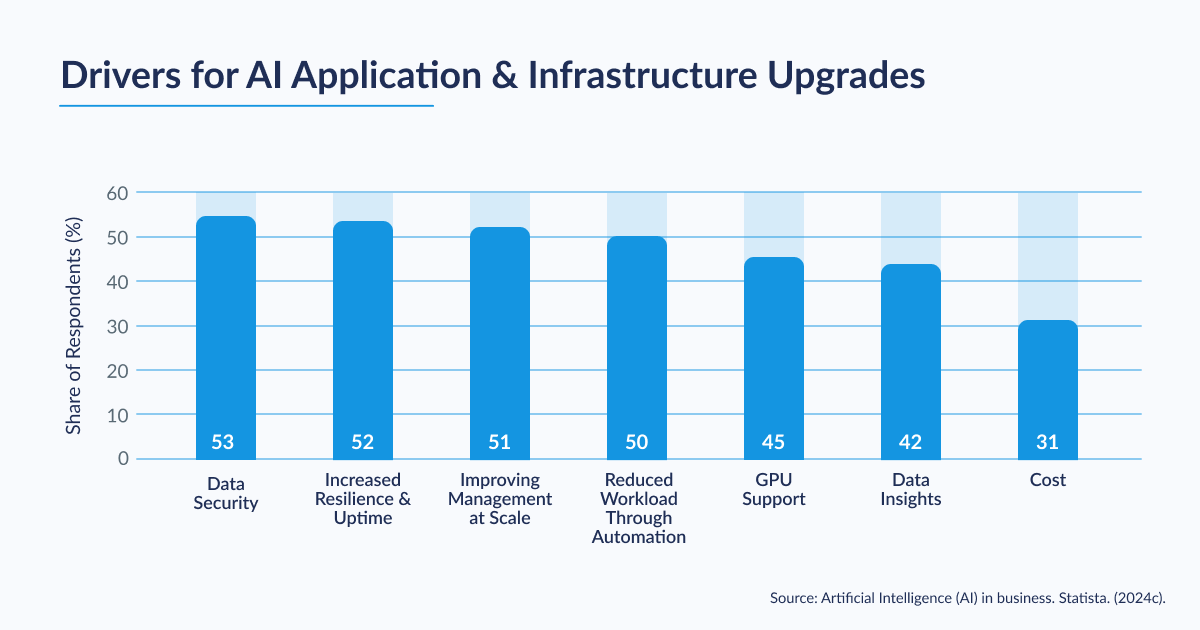
Given the dependence of efficient and effective AI operationalization on advanced data capabilities, the lack of data maturity across enterprises has emerged as a critical challenge and an all-too-frequent inhibitor to AI initiatives. Globally, more than 30% of companies cite data management as their leading infrastructure challenge regarding AI developments, and roughly 50% and 40% cite data security and data insights as their primary drivers for AI application and infrastructure upgrades, respectively. It’s also worth noting that Vation Ventures’ 2024 Technology Executive Outlook Report found that data and analytics was the third largest organizational skill gap reported by enterprise executives, further exacerbating the challenges arising from inadequate data maturity and technical talent constraints.

According to a recent survey of the Vation Ventures Technology Practitioner Council, quality and accuracy (79%) and security and privacy (79%) overwhelmingly represent the two most pressing challenges in AI data preparedness. These elements are foundational to a mature data management posture and, in turn, enable enhanced and aligned AI technology performance and security. As a result, enterprises are incurring significant and fundamental upstream data challenges that accentuate the need for improved data maturity and management practices, particularly regarding quality, security and analytics.
Model Development
The development and refinement of AI models present numerous challenges and considerations for enterprises aiming to operationalize AI effectively. Although there are specific nuances and considerations depending on open-source or closed-source approaches, enterprises face several critical decisions in building, developing, and operationalizing AI models and solutions. Generally, there are four foundational development layers, each containing various approaches, structures, and considerations. The following provides a high-level overview of these layers and some of the key corresponding approaches currently being utilized.
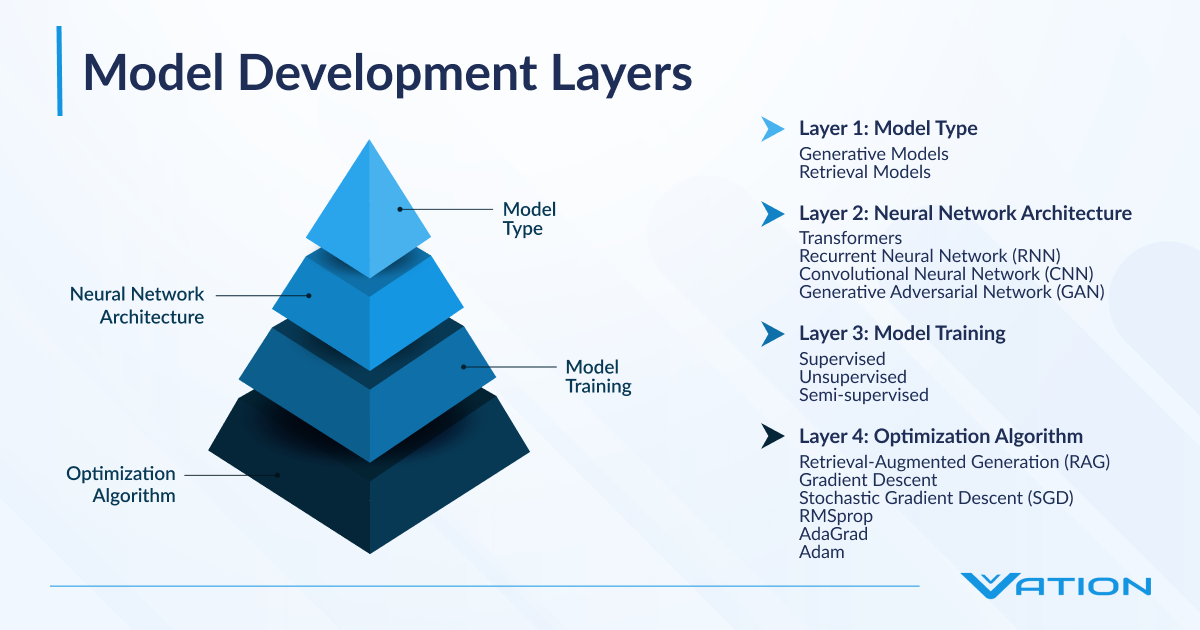
Layer 1: Model Type
This foundational layer defines the fundamental structure and design of the model. The model layer determines how the input data is processed and what kind of output is generated. Different model types are chosen based on the nature of the task, such as sequential data processing or predicting continuous values. The choice of model type impacts the overall performance and suitability for various applications.
- Generative Models: Generative models create new data samples based on the training data they have been exposed to. They are used in applications like image synthesis, text generation, and more, with several subtypes that offer unique advantages for specific tasks.
- Diffusion Models: Diffusion models generate data by iteratively refining noise until it matches the desired distribution. They excel in image and video generation tasks, providing high-quality and diverse outputs.
- Foundational Models: Foundational models are large, pre-trained AI models that serve as a base for various downstream tasks. They are trained on extensive datasets and can be fine-tuned for specific applications, offering a versatile starting point for AI development.
- Large Language Models: LLMs are designed to understand and generate human-like text by leveraging massive amounts of text data during training. They excel in natural language processing tasks, such as translation, summarization, and question-answering, due to their ability to capture nuanced linguistic patterns.
- Multimodal Models: Multimodal models integrate and process data from multiple modalities, such as text, images, and audio, to generate more comprehensive and accurate outputs. This capability makes them particularly useful in applications that require understanding and combining diverse data types.
- Retrieval Models: Retrieval models are designed to fetch relevant information from a large dataset based on a given query. They are essential in search engines and recommendation systems, which aim to provide precise and relevant results from vast information pools.
Layer 2: Neural Network Architecture
The neural network architecture layer specifies the structural design of the neural network used in the model. This includes the arrangement of layers, the flow of data, and the specific mechanisms employed for processing information. Different architectures are tailored to handle various data types and tasks, optimizing the model’s ability to learn and make accurate predictions.
- Transformers: Transformers utilize self-attention mechanisms and an encoder-decoder architecture to process data in parallel, making them highly efficient for natural language processing tasks. They excel in capturing long-range dependencies and contextual relationships in text and data, serving as the backbone for many leading AI model solutions.
- Recurrent Neural Network (RNN): RNNs are designed for sequential data, maintaining a hidden state that captures information from previous inputs. They are well-suited for tasks like time series prediction and language modeling.
- Convolutional Neural Network (CNN): CNNs apply convolutional filters to input data, making them highly effective for image and spatial data processing. They are widely used in computer vision applications for image recognition and object detection tasks.
- Generative Adversarial Network (GAN): GANs consist of a generator and a discriminator that compete to create realistic data samples. They are popular for generating high-quality synthetic data, such as images and videos.
Layer 3: Model Training
Model training involves teaching the neural network to recognize patterns in data through various learning methods. The choice of training method impacts how well the model generalizes to new data and its overall performance. Training can be supervised, unsupervised, or semi-supervised, each with advantages and use cases.
- Supervised: The model is trained on labeled data in supervised learning, making it effective for tasks where the output is known. Regression predicts continuous values within supervised learning, while classification categorizes data into predefined classes.
- Unsupervised: Unsupervised learning involves training on unlabeled data to discover hidden patterns and structures. Within unsupervised learning, clustering is used to group similar data points together, while association finds relationships between variables in large datasets.
- Semi-supervised: Semi-supervised learning combines a small amount of labeled data with a large amount of unlabeled data. This approach leverages the advantages of both supervised and unsupervised learning, improving model performance with smaller amounts of labeled data.
Layer 4: Optimization Algorithm
The optimization algorithm layer focuses on improving the model’s performance by adjusting its parameters to minimize errors. Different algorithms are used to efficiently navigate the complex landscape of the model’s loss function. Choosing a suitable optimization algorithm is crucial for improving convergence and performance.
- Retrieval-Augmented Generation (RAG): RAG combines generative models with information retrieval, enhancing a model’s ability to generate accurate and relevant responses by pulling information from a large dataset.
- Gradient Descent: Gradient descent is a fundamental optimization algorithm that iteratively adjusts model parameters to minimize the loss function. It is widely used for its simplicity and effectiveness in various machine-learning tasks.
- Stochastic Gradient Descent (SGD): SGD updates model parameters using a randomly selected subset of data points, making it faster and more efficient for large datasets. This optimization approach helps reduce computation time and improve convergence speed.
- RMSprop: RMSprop adjusts the learning rate based on the average of recent gradients, preventing oscillations and speeding up convergence. It is particularly useful for training deep neural networks with non-stationary objectives.
- AdaGrad: AdaGrad adapts the learning rate for each parameter based on its historical gradients, allowing for more significant updates for infrequent parameters. This method effectively deals with sparse data but may suffer from diminishing learning rates over time.
- Adam: Adam is an adaptive learning rate optimization algorithm that combines the benefits of AdaGrad and RMSprop. It is well-suited for handling sparse gradients and noisy data and provides robust performance across different tasks.
Responsible Governance
The rapid advancement of AI technologies has led to the emergence of responsible AI governance, which is grounded in principles of transparency, explainability, accountability, fairness, and security. According to Vation Ventures’ 2024 Technology Executive Outlook Report, 64% of global CXOs plan to implement responsible AI, making it the top emerging technology focus area. Effective governance frameworks are crucial for harnessing the transformative potential of AI technologies while ensuring they are used safely and securely. These frameworks enable enterprises to align AI initiatives with external frameworks, such as regulatory or industry requirements, and internal business frameworks, enhancing their operational effectiveness and overall enterprise value.
The need for robust and responsible AI governance systems has been underscored by the poor performance of many major AI models on transparency indices and the absence of unified standards for benchmarking responsible AI performance. Despite their transparency and flexible customization advantages, open-source models can introduce security risks and vulnerabilities and underperform closed-source models in areas like coding, mathematical reasoning, and agent-based behavior. That said, most models, regardless of type, exhibit significant transparency gaps, particularly in upstream segments, including data sources, computing and labor, as well as in downstream segments, such as feedback and impact measures. These deficiencies undermine model effectiveness and complicate governance efforts, necessitating continuous monitoring and improvement.
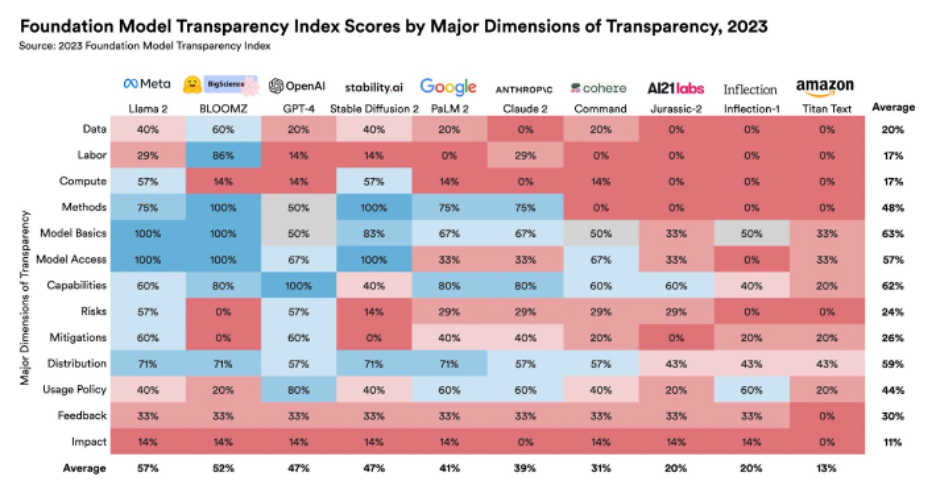
Regulatory bodies worldwide are implementing new policies and frameworks to govern AI development and use, aiming to balance innovation with accountability. These frameworks focus on privacy protection, data security and ethical standards, and influence how enterprises develop, market and deploy AI products. As these regulations evolve, enterprises and AI providers must develop compliance practices, allocate resources for regulatory monitoring, and invest in training and enablement to stay aligned with the regulatory landscape.
Enterprise AI Operationalization Roadmap
Based on the key enterprise challenges, the following serves as a strategic roadmap and framework for efficient and effective AI operationalization. Broken into six primary phases, the first three focus on enterprise assessment, planning, AI posture and enablement, whereas the last three focus on development, implementation and integrated deployment. By following and executing this six-phased AI roadmap, enterprises can more effectively and efficiently achieve AI operationalization to drive transformative value, growth and differentiation.

Phase 1: Assessment
Overview
The first and perhaps most important phase of the AI operationalization roadmap focuses on a thorough assessment of the current AI ecosystem within the enterprise. This phase is crucial for identifying gaps in capabilities, resources and infrastructure that may impede the successful implementation of AI initiatives. By conducting a comprehensive evaluation, organizations can set realistic goals, allocate resources effectively and develop a clear and tailored roadmap for achieving their AI objectives. This upfront assessment is vital in ensuring alignment with business goals and regulatory requirements, enhancing the likelihood of successful AI integration and value realization.
Elements & Considerations
- AI Readiness Assessment: This involves evaluating the maturity of the data infrastructure, technical capabilities and the existing support systems required for AI deployment. Understanding these elements is critical for identifying current strengths and weaknesses and planning the necessary upgrades and investments to build a robust and supportive AI ecosystem.
- Current & Future State: Assessing the current state of AI implementation and the desired future state helps create a realistic and achievable AI strategy. This understanding aids in setting clear milestones and objectives, ensuring that the transition toward advanced AI capabilities is structured and measurable.
- Elemental Evaluation: This involves thoroughly analyzing various factors, including technical infrastructure, data maturity, business processes, operational workflows and regulatory constraints. By considering all these elements, enterprises can develop a holistic view of the challenges and opportunities, enabling them to craft a strategic plan that aligns with their AI goals and broader organizational objectives.
Phase 2: Planning & Mapping
Overview
The second phase of the AI operationalization roadmap involves meticulous planning and mapping to ensure the successful execution of AI initiatives. Here, the insights gained during the prior assessment phase are translated into a detailed strategic plan outlining the steps needed to achieve AI goals. Effective planning and mapping require a deep understanding of the potential impacts, clear communication and alignment with stakeholders, and the development of a measurable execution roadmap. By focusing on these elements, organizations can create a structured and actionable plan that drives AI operationalization and value realization.
Elements & Considerations
- Impact Analysis: Conducting a comprehensive impact analysis helps in understanding the technical, operational and financial implications of AI initiatives. This analysis enables enterprises to anticipate potential challenges, allocate resources efficiently, and develop a cost-effective implementation strategy. Organizations can improve decision-making, reduce risk and enhance operationalization efficiency and effectiveness by identifying and quantifying impacts.
- Stakeholder Engagement: Clear and consistent communication is crucial for aligning all stakeholders and securing buy-in for AI initiatives. Achieving this involves engaging with key decision-makers, technical teams and end-users to ensure everyone understands the benefits and objectives of the AI strategy. Building consensus and fostering a collaborative environment helps mitigate resistance to change, improves operational efficiency and enhances the overall success of AI initiatives.
- Execution Roadmap: Developing a detailed execution roadmap with tangible, aligned and measurable KPIs ensures that AI initiatives are tracked and evaluated effectively. Such a roadmap should outline specific milestones, timelines, and performance indicators that align with business objectives. By linking the execution plan to KPIs, organizations can more effectively monitor progress, make necessary adjustments and demonstrate the value of AI investments to stakeholders.
Phase 3: Tooling
Overview
The third phase of the AI operationalization roadmap focuses on selecting and implementing the right tools and technologies to support AI initiatives. This phase is crucial for establishing the infrastructure and processes required to effectively develop, deploy, and manage AI solutions effectively. Proper tooling ensures that AI systems are scalable, secure and governed responsibly while enabling organizations to accurately measure performance and ROI. By addressing these elements, enterprises can build a robust technological foundation that supports their AI goals and facilitates ongoing improvement, optimization and innovation.
Elements & Considerations
- Executional Accountability & Visibility: Defining clear roles and responsibilities is essential for the successful implementation of AI initiatives. This involves assigning specific tasks and accountability to team members, ensuring that all aspects of the AI project are managed, communicated and coordinated effectively. Additionally, establishing clear and visible process, business and operational metrics for ROI and performance measurement allows organizations to track the success of AI initiatives, make data-driven decisions and demonstrate the value of AI investments to stakeholders.
- Enablement & Support: Based on the specific AI maturity, posture and strategic roadmap, enterprises must identify and develop the necessary partnerships, expertise, tooling and support enablement to execute AI initiatives. This includes collaborating with technology providers and industry experts to access advanced tools and resources while prioritizing comprehensive internal training and enablement programs. By leveraging external expertise, internal enablement and robust support systems, organizations can accelerate AI operationalization and enhance their technical capabilities.
- Responsible Governance Alignment: Developing a responsible governance framework is vital for ensuring greater observability and explainability of AI systems. This framework should align with internal and external standards to mitigate risks and optimize performance. By incorporating robust security measures and adaptable governance structures, enterprises can maintain compliance, foster trust and enhance the overall effectiveness of their AI initiatives.
Phase 4: Development & Build
Overview
Following the first three planning and posture-oriented phases, the fourth phase of the roadmap turns to the actual development and building of AI solutions. This phase involves implementing the planned AI systems, ensuring they are secure, observable, scalable and adaptable to future needs. Effective development and build practices are essential for creating robust AI solutions that can seamlessly integrate into business operations. Organizations can ensure their AI initiatives are built on a solid foundation that supports long-term success and innovation by addressing specific elements such as security, observability and scalability.
Elements & Considerations
- AI Risk Management: Implementing and integrating a comprehensive AI risk management strategy is crucial for identifying and mitigating potential risks associated with AI deployment. This includes assessing data security, managing software supply chain vulnerabilities, ensuring compliance with privacy regulations and establishing robust guardrails to protect against ethical and operational risks. A proactive approach to risk management ensures that AI systems are resilient, secure and compliant with regulatory standards.
- End-to-end Observability: Ensuring comprehensive observability across an entire AI system is essential for maintaining its secure reliability and performance. This includes monitoring upstream data inputs and downstream outputs to promptly identify and address any issues. End-to-end observability enables organizations to track AI system behavior, optimize performance and make informed decisions based on real-time data insights.
- Scalability and Adaptability: Designing AI systems that prioritize scalability and adaptability is critical for future developments and changes. Doing so involves integrating data sources seamlessly, ensuring the system can scale efficiently, and optimizing performance and resource utilization. By prioritizing scalability and adaptability, organizations can ensure their AI solutions remain effective and dynamic as business needs and technologies evolve.
Phase 5: Piloting & Refinement
Overview
The fifth phase of the AI operationalization roadmap focuses on piloting and refining AI solutions before full-scale deployment. This phase is critical, for it involves validating the AI solution in real-world environments, gathering feedback and making necessary adjustments to ensure optimal performance and alignment with business objectives. By conducting targeted pilot rollouts, measuring and reporting performance, and continuously testing and refining the models, organizations can enhance the effectiveness and reliability of their AI initiatives, paving the way for successful large-scale implementation.
Elements & Considerations
- Intentional Pilot Rollouts: Conducting targeted pilot rollouts allows organizations to introduce AI solutions incrementally, providing channels for collecting feedback on usability, efficiency and effectiveness. This feedback is invaluable for identifying areas in need of optimization and improvement, which helps to ensure the AI system is well-tuned before broader deployment. Highly targeted and intentional rollouts help mitigate risks and ensure issues are addressed early in the process.
- Measurement & Reporting: Measuring and reporting the performance, impacts and findings of AI testing and piloting to stakeholders is essential for transparency and accountability. This involves tracking and communicating KPIs, business impacts and outcomes, challenges, insights and opportunities. Effective measurement and reporting enable stakeholders to understand the value and impact of AI initiatives, fostering greater support and alignment.
- Testing & Refinement: Continual testing and refinement of AI solutions are crucial for achieving optimized alignment with business goals and ensuring the system’s readiness for expanded rollout. This process involves iterative testing to identify and fix issues, enhance functionalities, and fine-tune the model based on real-world performance data. By prioritizing ongoing refinement, organizations can ensure their AI solutions are robust, reliable and capable of delivering sustained value as they scale.
Phase 6: Deployment & Integration
Overview
The final phase of the AI operationalization roadmap involves the full-scale deployment and integration of AI solutions into the enterprise’s technological and operational environment. This phase ensures that AI systems are seamlessly integrated and that they function effectively within the existing infrastructure, operational workflows and technology ecosystem. By focusing on systematic integration, continuous monitoring and optimization, and the exploration of accretive opportunities, organizations can maximize the benefits of their AI initiatives and drive sustained growth and innovation.
Elements & Considerations
- Systematic Integration: A full, expanded and full rollout involves integrating AI solutions within the existing technological and operational environment. This process ensures that AI systems work harmoniously with current assets and processes, minimizing disruption and maximizing efficiency. Systematic integration is critical for leveraging the full potential of AI technologies across various functions and departments within the organization.
- Continuous Monitoring & Optimization: Monitoring AI performance, security, and resource utilization is essential for maintaining and enhancing system efficiency and effectiveness. By ensuring automated, real-time tracking of AI operations, enterprises can quickly and efficiently identify potential issues and adapt to rapidly evolving technological, operational, business, social and regulatory dynamics, driving greater AI optimization and performance.
- Accretive Opportunities: Leveraging the deployed AI solution and the broader AI ecosystem developed for additional expansion opportunities can drive competitive differentiation, operational automation and innovation-fueled growth. By identifying areas where AI can further enhance business processes or create new capabilities, organizations can continue to innovate and stay ahead of the competition. Accretive opportunities allow enterprises to capitalize on their AI investments, fostering an environment of continuous improvement and strategic advancement.
Conclusion
While transformative value realization is the ultimate goal of enterprise AI initiatives , the path to operationalization is often fraught with expected and unexpected challenges, complexities and nuances. As a result, enterprises have a tendency to stumble and fall throughout the journey, incurring costly delays, expenditures and setbacks that can and do lead to initiative termination. Enterprises must prioritize cultivating a more robust and mature AI posture and ecosystem to truly harness and operationalize the untapped potential and opportunity offered by AI technologies. To navigate AI operational challenges effectively, organizations must adopt a highly detailed, strategic roadmap that addresses the multifaceted aspects of AI planning, development and implementation. This approach is essential for mitigating risks, optimizing performance and ensuring AI projects deliver their intended value.
Looking to maximize the potential of your AI initiatives? Contact Vation Ventures today to explore our AI Advisory & Consulting Services. Whether you need comprehensive AI Governance as a Service, expert guidance on AI Use Case Prioritization, or a tailored AI Strategy, our advanced solutions and industry insights will help you navigate the complexities of AI operationalization. Reach out to our experts and Research Team now to ensure your AI projects are ethical, compliant, and strategically aligned for maximum ROI and competitive advantage.