Foundations for AI Success: Navigating Data Preparedness and Large Language Model Selection in 2024
Artificial intelligence is a top priority for most businesses, and organizations are now grappling with the challenges of laying a solid foundation for successful AI initiatives. Our recent survey of the Innovation Advisory Council explores the heart of AI readiness, discovering the intricate challenges that companies face in data preparedness, quality improvement, and large language model (LLM) selection. The Innovation Advisory Council is an exclusive global network of over 1,000 leading technology executives who meet to share insights and build partnerships while gaining exposure to and advising early-stage companies. Innovation Advisory Council members collaborate on real world challenges to not only drive their own company’s innovation but to help shape the technologies of tomorrow.
By targeting key decision-makers, we sought to uncover the most pressing obstacles and strategic approaches shaping the AI journey for modern enterprises. The insights gathered reveal a complex ecosystem of technical, organizational, and strategic considerations that can make or break the potential of AI initiatives.
Tackling Data Challenges to Unlock AI Potential

Data is the cornerstone of any successful AI initiative, serving as the foundation upon which AI models are built and insights are derived. As organizations continue to embrace AI in their operations, the importance of being data-ready is more crucial than ever. High-quality, accurate data is essential for reliable outcomes, yet challenges such as data integrity, security, and bias often impede progress. Leaders must prioritize robust data management practices, from ensuring accuracy and security to addressing ethical concerns like bias and fairness. By overcoming these challenges, organizations can unlock the full potential of AI, driving innovation, informed decision-making, and a sustainable competitive edge.
- Data Quality and Accuracy: With 79% of leaders citing quality and accuracy as top challenges in data preparedness for AI, this highlights a critical issue for business technology executives. High-quality, accurate data forms the backbone of successful AI implementations. Leaders must ensure rigorous data collection processes and validation mechanisms are in place to maintain data integrity. Data quality can lead to better insights, undermining AI initiatives. Thus, prioritizing data accuracy is vital for using AI to drive informed decision-making and competitive advantage.
- Security and Privacy Concerns: A notable 79% of leaders cite security and privacy as significant challenges, underscoring the critical need to protect sensitive data in AI initiatives. As cyber threats and data breaches become increasingly prevalent, implementing robust security measures and stringent privacy protocols is more important than ever. Organizations must foster a culture of security awareness and invest in advanced technologies to safeguard data assets. Proactively addressing these concerns mitigates the risk of breaches and strengthens trust with customers and stakeholders, an essential factor in preserving business reputation and ensuring regulatory compliance.
- Data Cleaning and Preprocessing: Although only 29% of leaders see cleaning and preprocessing data as a challenge, it remains a crucial aspect of AI data preparation. This process involves transforming raw data into a usable format, which is essential for accurate AI model training. Leaders should emphasize investing in automated tools and skilled personnel to streamline these tasks. Efficient data preprocessing ensures that AI models are trained on reliable data, leading to better performance and more reliable outcomes.
- Addressing Bias and Fairness: Similarly, 29% of leaders are concerned with bias and fairness in AI data, underscoring the need for ethical AI practices and data quality. Ensuring that AI systems are free from bias and operate fairly is critical for maintaining public trust and avoiding legal repercussions. Leaders must implement practices to identify and mitigate bias, such as diverse training data and algorithm audits. By proactively addressing these issues, businesses can develop AI solutions that are equitable and just, reinforcing commitments to responsible AI use.
Data readiness is the foundation of successful AI initiatives, requiring a focus on quality, security, preprocessing, and ethics. Addressing challenges like accuracy, security risks, and bias ensures reliable AI outcomes and builds trust with customers and stakeholders. Organizations can unlock AI’s full potential by adopting strong data management practices and ethical AI principles, driving innovation, better decisions, and a lasting competitive edge. As AI reshapes business, those prioritizing data readiness will lead the charge in the digital age.
Building a Strong Data Foundation for Ensuring Quality and Reliability
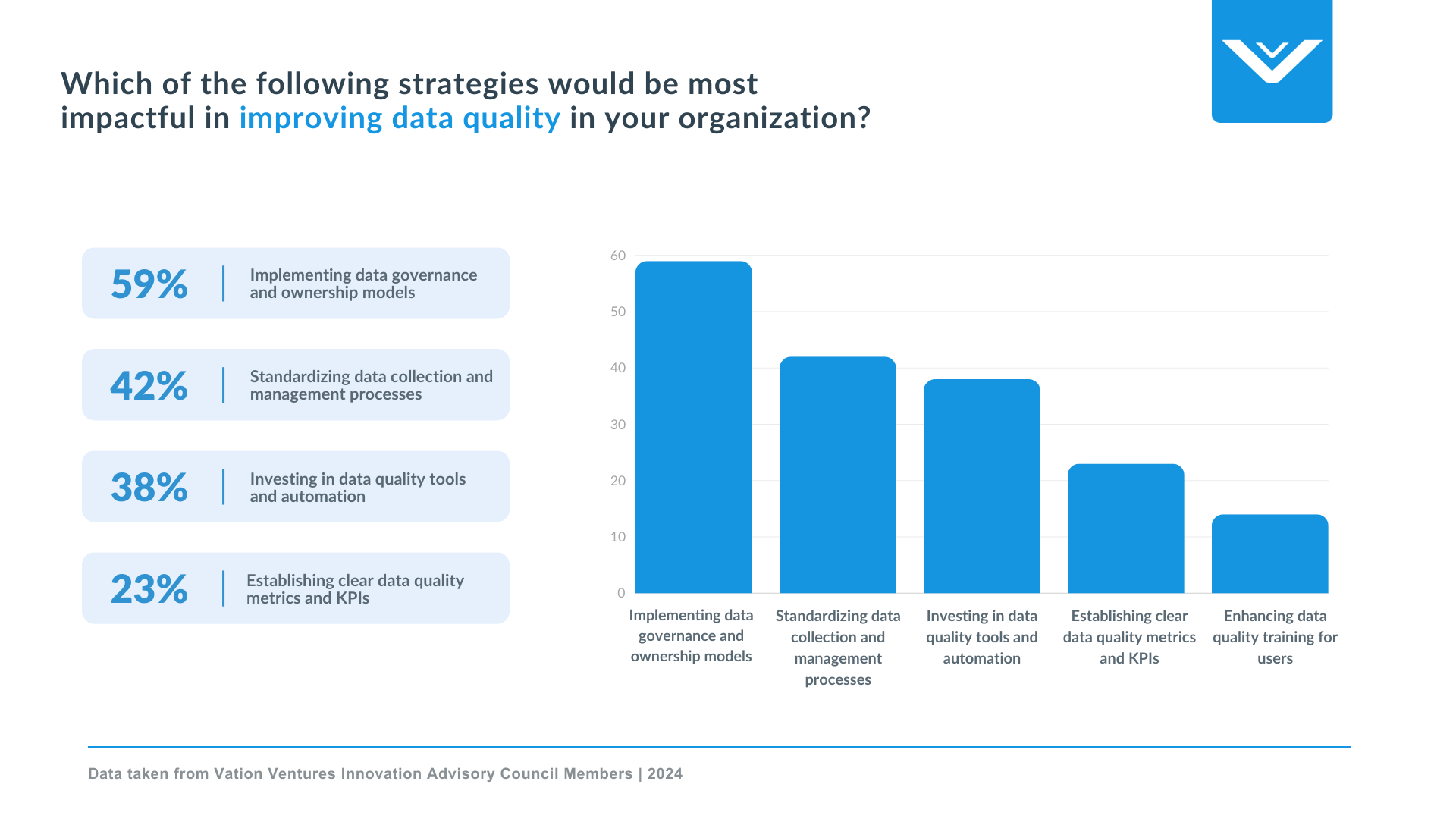
High-quality data is the cornerstone of successful AI initiatives, driving accurate insights, reliable analytics, and effective decision-making. However, ensuring data quality remains a significant challenge for many organizations. To support AI initiatives and maximize their potential, leaders must adopt strategies that strengthen data governance, standardize processes, and leverage advanced tools. These efforts ensure data is consistent, accurate, and accessible, providing the robust foundation AI systems need to deliver meaningful results. By addressing data quality challenges, organizations can unlock the full power of AI, driving innovation and maintaining a competitive edge in a rapidly evolving market. Here are three strategies, drawn from insights within our technology executive community, that leaders can use to enhance data quality in their organizations:
- Implementing Data Governance and Ownership Models: At 59%, most leaders believe that establishing data governance and ownership models is crucial for improving data quality. This strategy involves defining clear roles, responsibilities, and processes for managing data assets across the organization. Effective data governance ensures that data is consistently accurate, reliable, and accessible. It fosters accountability as stakeholders understand their roles in maintaining data quality. Leaders should focus on developing and enforcing policies that standardize data handling practices, thus reducing errors and enhancing data integrity, ultimately leading to more informed decision-making and strategic advantages.
- Standardizing Data Collection and Management Processes: 42% of leaders cite the standardization of data collection and management processes as the second most impactful strategy. This approach emphasizes uniformity in how data is gathered, stored, and utilized across the organization. By implementing standardized procedures, businesses can minimize discrepancies and ensure consistency in data quality. This enhances data reliability and simplifies data integration and analysis, facilitating a more cohesive and efficient data ecosystem.
- Investing in Data Quality Tools and Automation: Finally, 38% of leaders recognize the importance of investing in data quality tools and automation to improve data quality. These tools offer advanced data cleansing, validation, and enrichment capabilities, reducing the manual effort required and mitigating human error. Automation can significantly enhance the efficiency and accuracy of data management processes. Leaders should prioritize deploying sophisticated data quality solutions that continuously monitor and rectify data issues. By doing so, organizations can maintain high data quality standards, enabling more precise analytics and better business outcomes.
By prioritizing these strategies, technology leaders can effectively address data quality challenges. Implementing governance models, standardizing processes, and leveraging automation are critical steps in building a complete data foundation. This approach ensures that organizations can harness the full potential of their data assets, fostering innovation and driving meaningful outcomes.
Key Considerations for Selecting Large Language Models (LLMs)
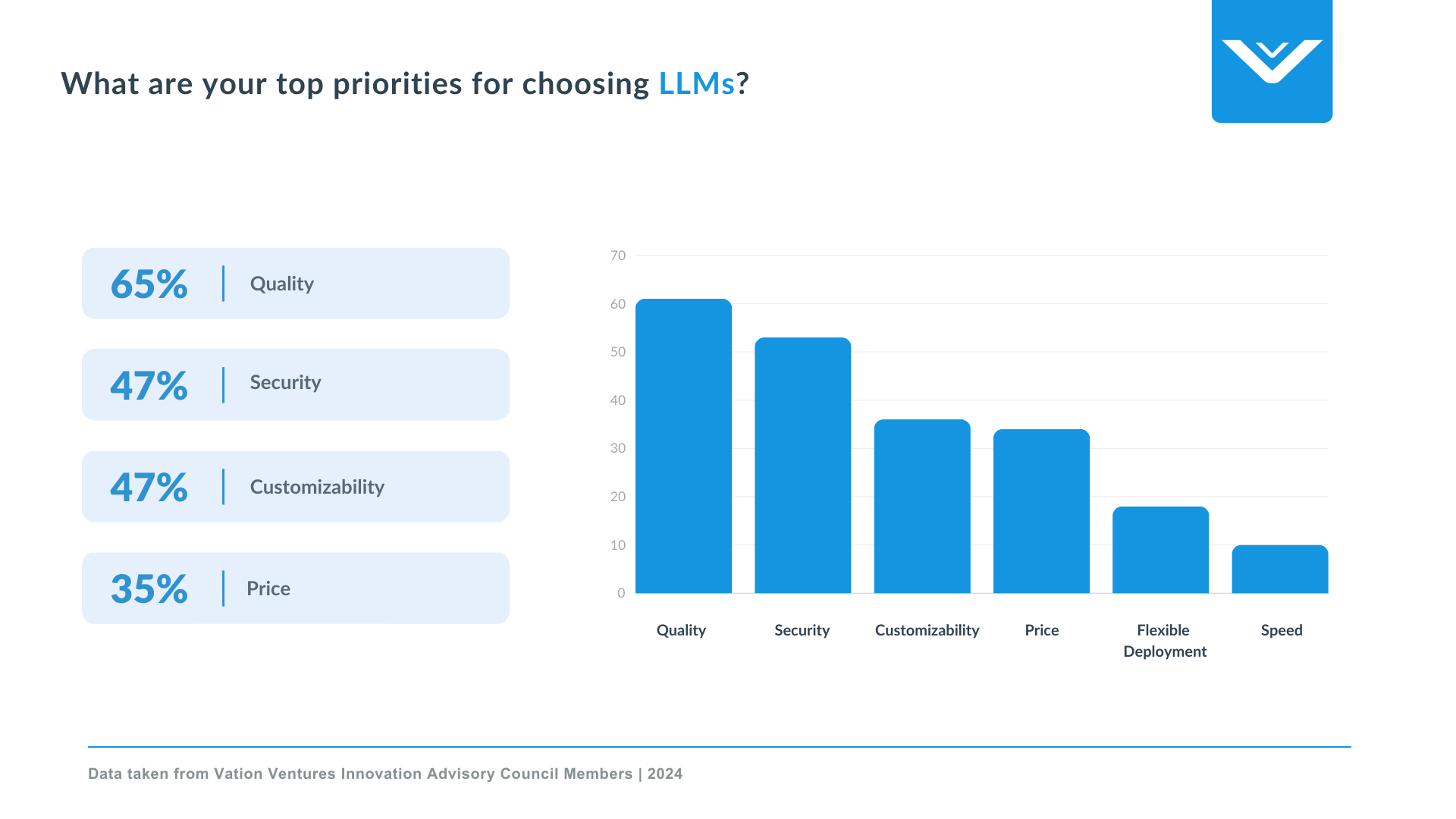
Selecting a suitable AI language model has become a pivotal decision for tech leaders. The choice isn’t solely about raw computational power but identifying a solution that aligns with the organization’s unique needs. Organizations assess AI models based on six essential factors: output quality, security, customization capabilities, cost, deployment flexibility, and responsiveness. The objective isn’t merely to adopt and leverage AI as a strategic advantage in today’s competitive business landscape.
- Quality: For 61% of leaders, quality is the top priority when choosing Large Language Models (LLMs). High-quality LLMs produce accurate, coherent, and contextually appropriate outputs, critical for applications ranging from customer service to data analysis. Leaders must evaluate LLMs based on their ability to generate reliable and relevant results consistently. Ensuring that the LLMs are trained on diverse and extensive datasets is essential for superior performance. Prioritizing quality in LLM selection helps organizations use AI to enhance decision-making, improve customer experiences, and drive operational efficiency.
- Security: Security is also a priority for 53% of leaders when selecting LLMs, highlighting the importance of protecting sensitive information and maintaining data integrity. Integrated into various business processes, LLMs must comply with stringent security standards to prevent unauthorized access and data breaches. Leaders should ensure that chosen LLMs incorporate vigorous encryption, secure data handling practices, and regular security audits. By focusing on security, organizations can safeguard their intellectual property and customer data, fostering trust and compliance with regulatory requirements.
Technology leaders can ensure their AI initiatives are effective and secure by emphasizing quality and security in LLM selection. High-quality LLMs enable accurate and meaningful interactions while detailed security measures protect valuable data. This balanced approach allows organizations to capitalize on AI advancements, enhancing performance and trust in AI-driven solutions.
Conclusion
The findings from the Innovation Advisory Council highlight the essential priorities for thriving in 2024 and beyond. Data preparedness, quality, and security are at the forefront, forming the foundation for successful AI applications. For technology leaders, focusing on high-quality, secure data and selecting robust Large Language Models is critical for training systems and delivering meaningful outcomes that align with core business goals. By embracing these strategies and fostering a culture of continuous improvement, executives can confidently navigate the evolving tech landscape, unlocking the full potential of AI to drive innovation and sustainable growth.
To navigate the complexities of AI implementation and make lasting changes within your organization, contact our AI experts for a customized roadmap tailored to your specific needs. By partnering with us, you can ensure that your organization is not only prepared for AI success but is also positioned to drive meaningful innovation and achieve a sustainable competitive edge in the evolving digital landscape. Don’t wait—reach out today to begin your AI transformation journey.