Innovation & Optimization: The Tightening AI Tightrope
The 2025 Technology Executive Outlook Report highlights a defining enterprise challenge: balancing the accelerating pace of innovation with the growing need for optimization, particularly regarding AI. While enthusiasm for AI remains high, the gap between ideation and implementation is widening due to barriers like talent shortages, data complexity, and technical trade-offs.
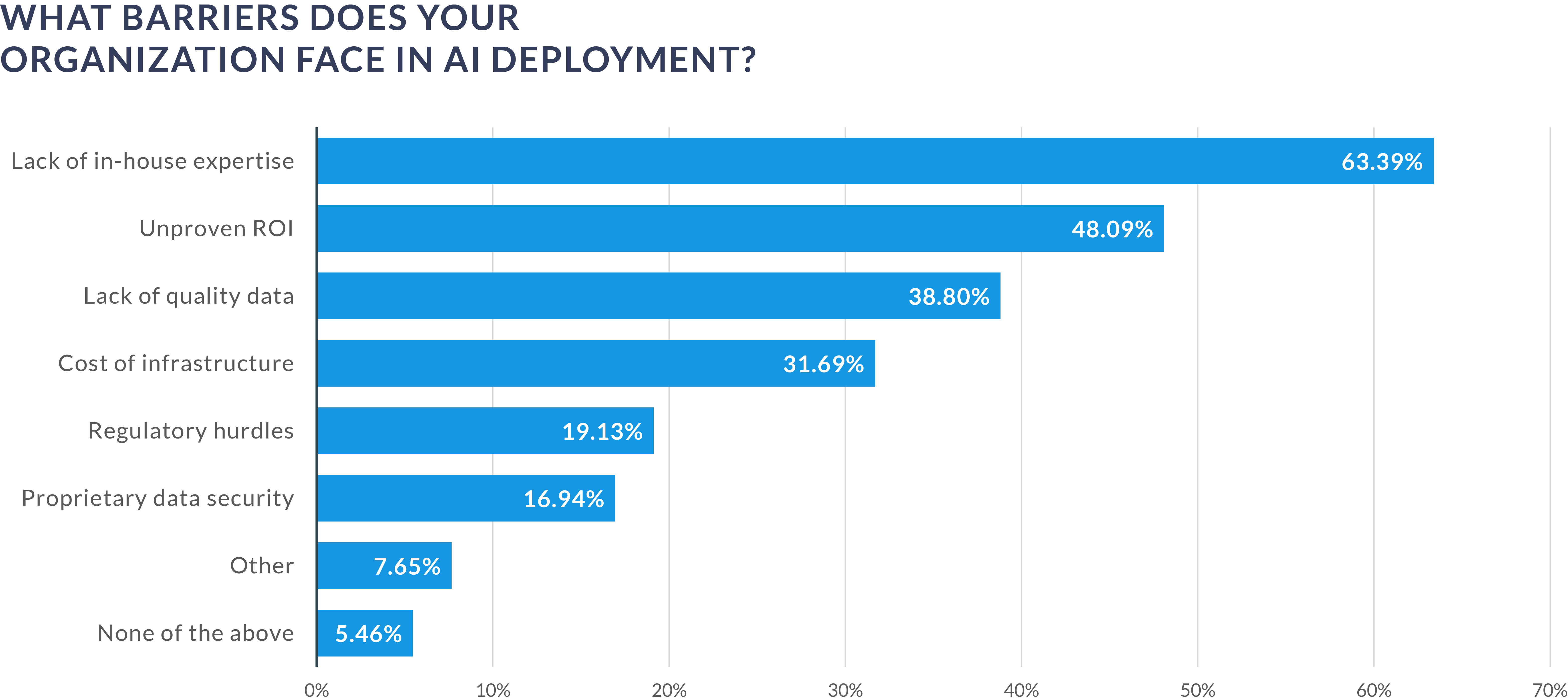
The surge in AI innovation has ignited excitement across C-suites, but executional reality remains more sobering. While 90% of technology executives cite AI as the most impactful emerging technology, more than 50% have yet to move beyond the planning stage and just 10% report full operationalization. The enthusiasm is real, but the operational gap—fueled by skills shortages, unproven ROI, and data quality issues—is even more pressing.
- The excitement around AI is unmistakable, but operationalizing it requires a precise balancing act between performance, scalability, governance, and real-world application. This means navigating a complex decision tree of technical and strategic trade-offs for many enterprises.
- Open-Source & Closed-Source Models: Open-source AI provides transparency and control for advanced teams, while closed-source models offer speed and ease of use, presenting a differentiated set of trade-offs between flexibility and operational efficiency.
- Aspirational & Functional Use Cases: Enterprises are finding early success by focusing on functional AI use cases that are small in scope and high in ROI, using these to build toward more advanced agentic and multimodal capabilities.
- Structured & Unstructured Data: Structured data supports faster and more reliable AI deployment, while unstructured data offers richer insights—but requires more mature infrastructure and governance to operationalize effectively.
- Data Modernization: The rise of data lakehouses reflects a shift toward unified data platforms that streamline access, governance, and performance across structured and unstructured data environments—crucial for scaling AI.
- Technical Talent & Accessibility: With AI as the top skills gap, enterprises are investing heavily in internal training and immersive, hands-on environments to build scalable talent pipelines and shorten time-to-proficiency.
Open-Source & Closed-Source Models
Open-source systems offer a clear advantage in flexibility, observability, and customization, empowering teams to tailor solutions to specific business needs. However, they demand a high level of internal technical expertise, significant investment in model fine-tuning and monitoring, and a higher burden of responsibility for security and reliability. Conversely, closed-source enterprise-ready models offer greater deployment speed and performance, reduced operational resourcing, and out-of-the-box functionality but come at the cost of limited control, customization, and transparency.
.png)
Aspirational & Functional Use Cases
While agentic AI and multimodal systems promise transformative capabilities, their operational complexity makes them unsuitable as starting points for most enterprises. To ensure more sustainable and optimized innovation, those lacking AI maturity and deep technical expertise should initially focus on functional workflow automation across small-scale and well-defined tasks. This not only provides immediate value realization and ROI, addressing one of the leading AI operationalization challenges, but also drives greater accessibility, safer experimentation, and a more secure foundational AI framework. Underpinned by a more intentional, pragmatic, and impact-driven focus, this basis enables an iterative and progressively more advanced, sophisticated, and aspirational AI operationalization roadmap process.
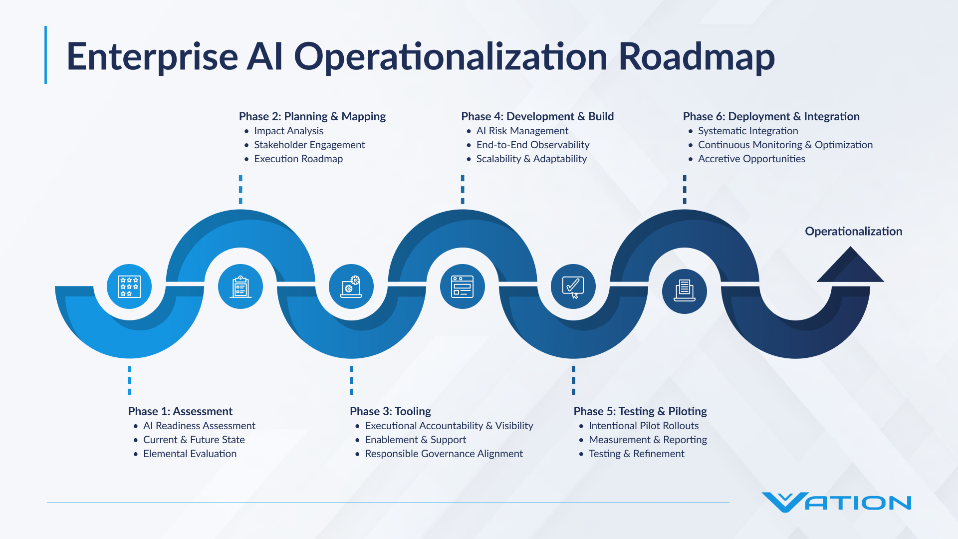
Structured & Unstructured Data
Structured data provides the most accessible and efficient foundation for early-stage AI operationalization. Found in systems like CRMs, ERP platforms, and industrial IoT sensors, structured data is inherently organized, well-labeled, and easier for AI models to process. It enables faster time-to-insight, lower resource overhead, and clearer performance measurement. By anchoring AI use cases in structured data, enterprises reduce complexity and create a stable baseline for innovation that’s measurable, governable, and optimized for ROI.
Unstructured data, on the other hand, introduces both complexity and opportunity. Rich in context but difficult to standardize, this includes formats such as text, images, video, and audio. Operationalizing unstructured data requires advanced NLP models, computer vision systems, and data pipelines built for high variability and ambiguity. As AI systems improve, their ability to understand and act on unstructured inputs will increasingly determine their value. However, for enterprises lacking high data maturity and technical depth, these use cases should be approached incrementally, driven by strong governance frameworks, observability mechanisms, and iterative deployment strategies to manage risk and ensure reliability.
Data Modernization
As enterprises scale AI and analytics initiatives, the structure and accessibility of their data environments become foundational to both innovation velocity and operational reliability. Traditional architectures—data warehouses for structured data and data lakes for unstructured storage—have long served distinct needs. Warehouses deliver the speed, reliability, and integrity required for real-time reporting and business intelligence. Lakes, on the other hand, offer the flexibility to store massive volumes of diverse, raw data—ideal for AI training and experimentation. However, managing both in parallel often leads to inefficiencies, duplication, and siloed pipelines.
Enter the data lakehouse: a hybrid architecture designed to unify the strengths of both lakes and warehouses in a single, scalable platform. By enabling the storage and processing of structured and unstructured data in one system, lakehouses reduce data redundancy, simplify data integration, and provide consistent governance across the full spectrum of data use cases. The modernized data fabric enabled by lakehouses streamlines ingestion, enriches context, and accelerates the operationalization of AI insights without compromising on quality or governance – two leading AI and data operationalization challenges.
.png)

Technical Talent & Accessibility
Technical talent and expertise remain one of the most persistent and practical barriers to AI operationalization. With 73% of enterprises citing AI as their leading skills gap, the issue is less about conceptual alignment and more about execution readiness. While hiring for specialized roles remains a piece of the puzzle, more than 70% of enterprises are prioritizing internal development and training as their primary path to closing the capability gap. This shift reflects a more sustainable and cost-effective strategy, one that prioritizes accessibility, institutional knowledge, and long-term workforce resilience over short-term expertise acquisition.
However, the road to proficiency and Future-Proofing IT isn’t instantaneous. Developing functional skills in new technologies typically takes 4–6 months, varying based on complexity and prior experience. There is growing effectiveness and momentum behind hands-on labs, sandboxes, and real-world simulation environments, as noted by more than 60% of enterprises, to accelerate upskilling and shorten time-to-impact. These immersive formats are proving more effective than traditional coursework, allowing employees to explore AI tools and frameworks in a safe, failure-tolerant environment.
.png)
Conclusion
The future of enterprise technology and the ability to effectively leverage transformative AI solutions will be directly shaped and influenced by the balance of increasingly fast-paced innovation and escalating pressure for optimization. AI’s potential is only realized when matched with the right data infrastructure, technical talent, and strategic frameworks, each requiring careful calibration rather than unchecked acceleration. Balancing innovation and optimization is not a static trade-off but an ongoing discipline: one that prioritizes clarity over complexity, intentionality over immediacy, and long-term scalability over short-term novelty. As we’ve seen, enterprises that master this balance will be best positioned to turn emerging technologies into enduring business value.
Looking to ensure the right balance between innovation and optimization across your AI initiatives? Contact Vation Ventures today to explore our AI Advisory & Consulting Services. Whether you need comprehensive AI Governance as a Service, expert guidance on AI Use Case Prioritization, or a tailored AI Strategy, our advanced solutions and industry insights will help you navigate the complexities of AI operationalization. Reach out to our experts and Research Team now to ensure your AI projects are ethical, compliant, and strategically aligned for maximum ROI and competitive advantage.