Data and Artificial Intelligence for Operational Efficiency: Technology Practitioner’s Perspective
While Data and Artificial Intelligence (AI) are each powerful forces on their own, bringing the two together can turn an enterprise technology laggard into a leader. If you’re old enough to remember the animated 1970-80s television series Super Friends, you might think of it in terms of that old refrain “Wonder Twin powers . . . activate!” If not, it’s sufficient to know that, in tandem, data and AI enable organizations to extract valuable insights, enhance decision-making processes and maintain a competitive edge in an increasingly data-driven world.
By prioritizing data and AI, organizations can mitigate risks associated with data mismanagement, streamline analytical processes and improve operational efficiency. For instance, robust data management and AI practices can support regulatory compliance while safeguarding sensitive information, as well as facilitate seamless integration with modern IT infrastructures and cloud environments. Further, the fusion of data and AI technologies can help organizations automate routine tasks, reducing manual oversight and allowing IT teams to focus more on innovation and strategic initiatives.
How Data and Artificial Intelligence Support Operational Efficiency
The effective use of data and artificial intelligence (AI) can boost operational efficiency by addressing core challenges and optimizing various business processes. AI-powered solutions can integrate data analysis into organizational workflows, automate routine tasks and enforce consistent data management policies. They can also speed informed decision making and support compliance initiatives. Some of the key benefits of pairing data and AI are:
Enhanced Decision-Making
Data and AI solutions enable organizations to make informed decisions by providing actionable insights derived from vast datasets. Approaches incorporating machine learning and predictive analytics can help identify trends and patterns, allowing businesses to forecast demand, optimize inventory and improve customer service. Real-time data analysis and AI-driven recommendations can also enable swift responses to market changes, thereby enhancing decision-making processes.
Improved Operational Efficiency
AI’s ability to automate business processes can go a long way toward reducing manual workloads and minimizing human error. Automated data processing, predictive maintenance and intelligent scheduling are but three examples of data-and-AI-based techniques for streamlining operations and freeing up resources for more strategic tasks. In addition to augmenting operational efficiency, they can ensure that business processes are applied consistently across an organization, allowing teams to spend more time on innovation and strategic initiatives.
Regulatory Compliance and Risk Management
Data-and-AI-based tools can support compliance with industry regulations and standards (GDPR, HIPAA, PCI-DSS, etc.) by providing comprehensive audit trails and reporting capabilities. Such tools can also enforce policies designed to help organizations meet regulatory requirements, such as data protection and privacy controls. By automating compliance-related tasks and maintaining detailed records of data activities, AI-powered data solutions can help organizations manage risks effectively and avoid costly penalties for non-compliance.
What Technology Practitioners Are Saying About Data and Artificial Intelligence
Though the benefits to be realized by fusing data and AI can give organizations a competitive edge, challenges remain. In fact, a recent survey fielded by Vation Ventures, revealed that technology practitioners have several critical challenges when it comes to preparing data for AI applications. Among the most significant has to do with the quality and accuracy of data, which 79% of respondents highlighted as a concern.
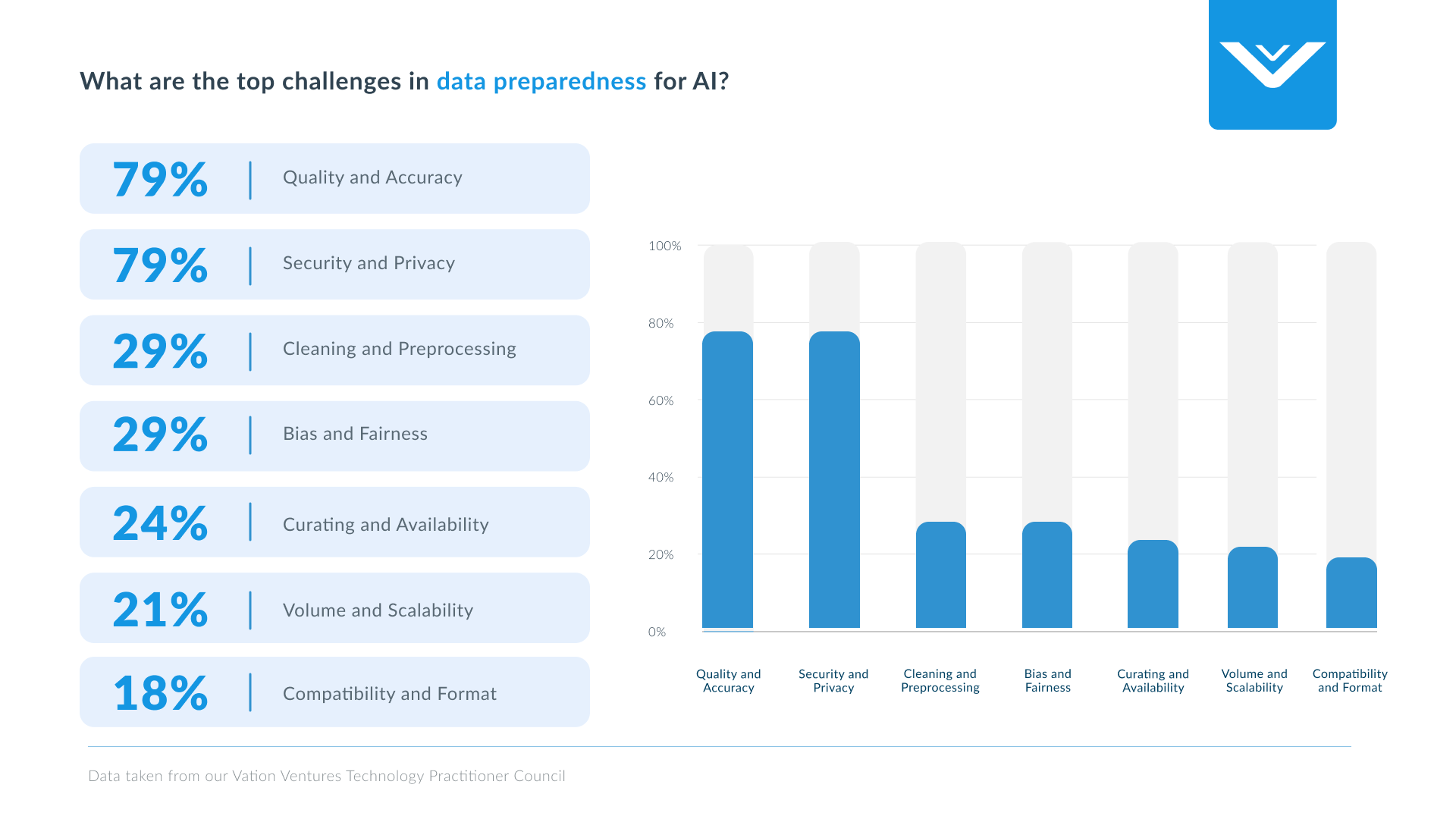
Ensuring that data is accurate and of a high quality is fundamental for the success of AI models, as flawed data can lead to incorrect insights and poor decision-making. Additionally, 79% of practitioners also pointed to security and privacy as major challenges. Safeguarding sensitive data from breaches and ensuring privacy compliance are crucial in maintaining trust and protecting organizational assets.
Another notable challenge has to do with data cleaning and preprocessing, which 29% of practitioners cited as a significant issue. These crucial tasks resolve errors in data, account for missing values and transform data into a suitable format for analysis, boosting the reliability of AI models (uncleaned data can significantly degrade model performance). What’s more, the fact that 29% of respondents also pointed to the challenge of bias and fairness as they relate to data and AI underscores the importance of ensuring that AI systems are trained on unbiased data to avoid perpetuating existing prejudices.
To address these challenges, technology practitioners identified several impactful strategies in our survey. The most favored approach, as chosen by 59% of respondents, is that of implementing data governance and ownership models. To be sure, establishing clear data ownership and governance frameworks helps ensure accountability and consistency in data management practices.
Investing in data quality tools and automation is another leading strategy for data quality improvement and was identified as such by 38% of respondents. These tools can automate the detection and correction of data issues. Additionally, standardizing data collection and management processes (42%) and establishing clear data quality metrics and KPIs (22%) are essential for maintaining high data quality standards.

Best Practices for Data and Artificial Intelligence
Vation Ventures advises enterprises to systematically evaluate and subsequently enhance their data and AI strategies to maximize efficiency and innovation. Organizations can identify areas for improvement and strengthen their data infrastructure by conducting thorough assessments and implementing a layered approach to data management and AI deployment, leveraging automation and detailed data governance plans, and cultivating a data-first culture that emphasizes collaborative intelligence. More specifically, best practices for data and AI include:
Strategic Assessment and Multi-Layered Data Strategy
Enterprises should start by systematically assessing their data needs through regular audits, quality checks and analytics to uncover potential gaps and opportunities. Following the assessment, it is essential to implement a multi-layered data strategy, which involves deploying various data management controls such as data encryption, access controls and data masking at different layers of the IT infrastructure. Finally, robust data governance frameworks and clear data are crucial to achieving accountability and consistency in data practices and – along with the other strategies already highlighted – can be achieved by investing in comprehensive data management solutions and continuously updating and testing data policies to adapt to evolving business requirements.
Automation and Data Quality Management
Automation significantly enhances data management by reducing manual workload and minimizing human error. Organizations can automate data processes using tools like data quality management (DQM) systems, which monitor data quality, detect anomalies, and rectify errors in real time, as well as through automated data integration and cleaning processes, which ensure that data remains accurate and consistent across different systems. A robust data quality management plan with procedures for maintaining data quality, including regular data audits and quality checks is also crucial, as are regular training and simulation exercises for data management teams and stakeholders to promote preparedness for such checks.
Culture of Data-First and Collaborative Intelligence
Fostering a data-first culture within an organization involves educating employees about data management best practices, such as recognizing data quality issues and using data responsibly, via regular training and awareness programs. Additionally, leveraging collaborative intelligence services and engaging with industry peers can help organizations stay updated on emerging data practices, enhancing their ability to manage and utilize data effectively. By implementing comprehensive training programs and engaging with external data communities to stay ahead of potential data challenges, organizations stand to improve their overall data management posture.
Conclusion
Clearly, the combination of data and AI plays a significant role in enhancing operational efficiency and innovation across the enterprise. The effective implementation of data management and AI solutions can establish a strong foundation for informed decision-making, boosting operational efficiency and strategic capabilities by streamlining processes, reducing risks associated with data mismanagement and improving the accuracy of predictive models. Data and AI also support regulatory compliance, protect sensitive information, and integrate seamlessly with modern IT infrastructures and cloud environments.
Looking ahead, the increasing complexity and volume of data will necessitate robust data management and AI practices for organizations seeking to maintain a resilient and adaptive operational environment. Integrating advanced data analytics and AI tools addresses challenges related to data quality, scalability and security, while prioritizing solutions with strong integration capabilities and compatibility with enterprise applications and legacy systems will be essential for maintaining cohesive data management practices and efficient operational workflows. Further, organizations that invest in comprehensive data and AI strategies will be better equipped to harness the full potential of their data, comply with regulatory requirements and adapt to the evolving technological landscape, ensuring long-term business continuity, stability and a competitive edge.
Our Technology Practitioner Council brings together directors and managers in technology focused on specific sectors, including Data & AI. The Council meets quarterly to discover emerging technologies in the space and hear exclusive trends from our Research team. Interested in joining this esteemed group? Get in touch today.